Data chaos instead of progress
Whether it's recipes, test results or process data — anyone who wants to make decisions in R&D often searches first: across folders, files and tables. Instead of structured collaboration and a clear overview, fragmented information, manual routines and double work characterize everyday life. Data is there — but not where it is needed.
Knowledge distributed
The results of previous tests are spread across network drives, Excel files, or emails. If you search, you lose time and repeat what has been known for a long time.
Data difficult to compare
Different formats, units and names make evaluation difficult. If you want to compare, you need time, contextual knowledge and often: a detour via Excel.
Administration instead of value creation
What could run automatically is often still done by hand. From data collection to reporting. This costs time without contributing to development.
Islands instead of integration
Data and processes run in separate tools, intermediate states and formats - without connection, without context, without a continuous flow of information.
All development steps at a glance. From formulation to result.
The right solution for every development team.
LabV is suitable for small teams as well as complex development environments. The platform replaces Excel chaos and individual solutions with a structured work environment in which data, tests and projects come together in a meaningful way. This reduces effort, accelerates development processes and improves decisions on a reliable basis.
Automated data import from test equipment
Import measurement values directly from devices - without manual transfer, without the hassle of formatting.
AI-powered development
The AI assistant reads data, understands content, performs calculations and helps with analysis. For faster answers and better decisions.
Central database for all projects
Projects, formulations, results and parameters in one place. Structured, searchable, versioned.
Digital collaboration
Test processes, results and projects can be documented directly in the system with comments, comments, tags and individual rights. In this way, everything remains comprehensible and in the right context.
Automated processes
Control tests, tests and approvals digitally - with templates and automated steps.
Open interfaces
Connect ERP, databases, or your own tools - for seamless integration and consistent data flows via API.
Better data. More efficient development.
Many R&D teams are considered digitized. But they search for test data in Excel spreadsheets, transfer measurement results manually and start projects over and over again. LabV starts at a different point: Instead of just documenting processes, our platform creates structure, context and access to networked knowledge. This turns isolated data into a learning system and research into a real competitive advantage.
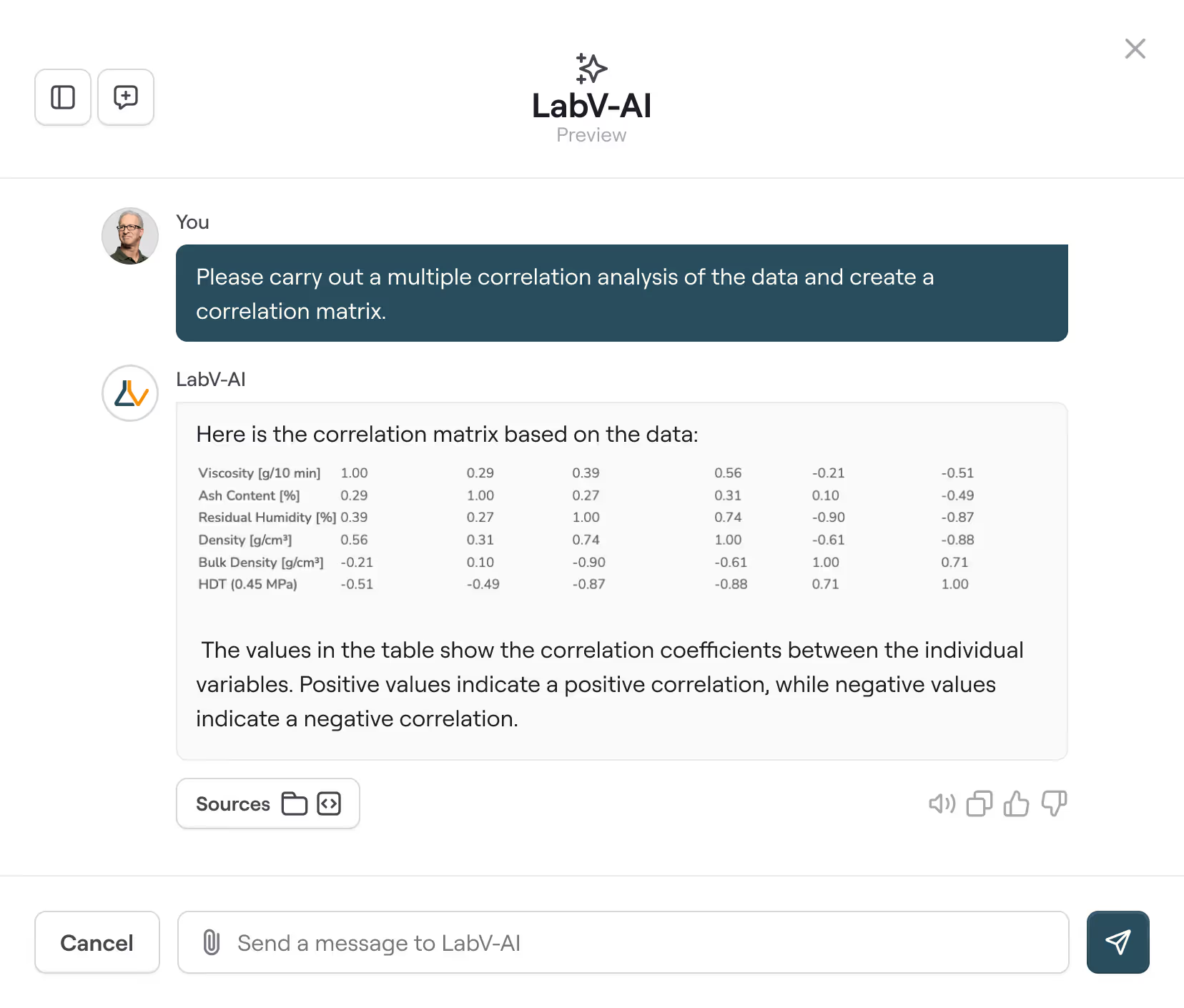
There is so much potential in your data
Use real figures to see how clearly structured data, less coordination and better comparability mean that development does not start from scratch, but leads to usable results more quickly.
Fewer repetitions: Relevant data can be found in a structured way and can be compared in the right context rather than spread across files, tables and headers.
More efficiency through less coordination and manual transfer. And therefore more time for what counts: development.
Our customers report impressive results
Common questions
Here's everything you need to know before you get started. Anything still unclear? Write to us
Our prices are flexible according to your needs and the size of your laboratory. You can test LabV free of charge and without obligation for 14 days — without installation costs or payment details.
Yes LabV meets the highest security standards: GDPR-compliant, with hosting on German servers, regular backups and encrypted data transmission. Your data belongs to you — anytime.
LabV can be easily integrated into existing LIMS, ERP and database systems — thanks to an open API and common interface standards. We would be happy to review your system landscape with you in advance.
LabV is immediately ready to go — no installation required. The intuitive user interface allows you to get started quickly without lengthy training. On request, we can provide you with personal onboarding and support.
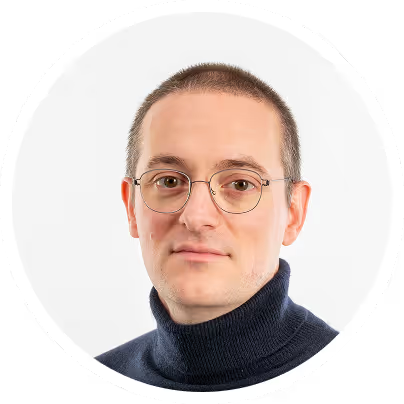


Test what really counts
In a free 45-minute demo session, experience how LabV makes complex processes simple, effortlessly combines data and makes everyday laboratory life noticeably easier with an intuitive interface.